Publications
AI FAQS: Key Concepts
Report Summary
We have created Frequently Asked Questions (FAQs) about the use of AI in financial services to create a resource for financial services stakeholders. These are designed for broad, non-technical audiences. The FAQs share our insights on how AI is being used in financial services and introduce key technological, market, and policy issues related to those applications. This first edition of our FAQs addresses a range of introductory questions about AI and machine learning.
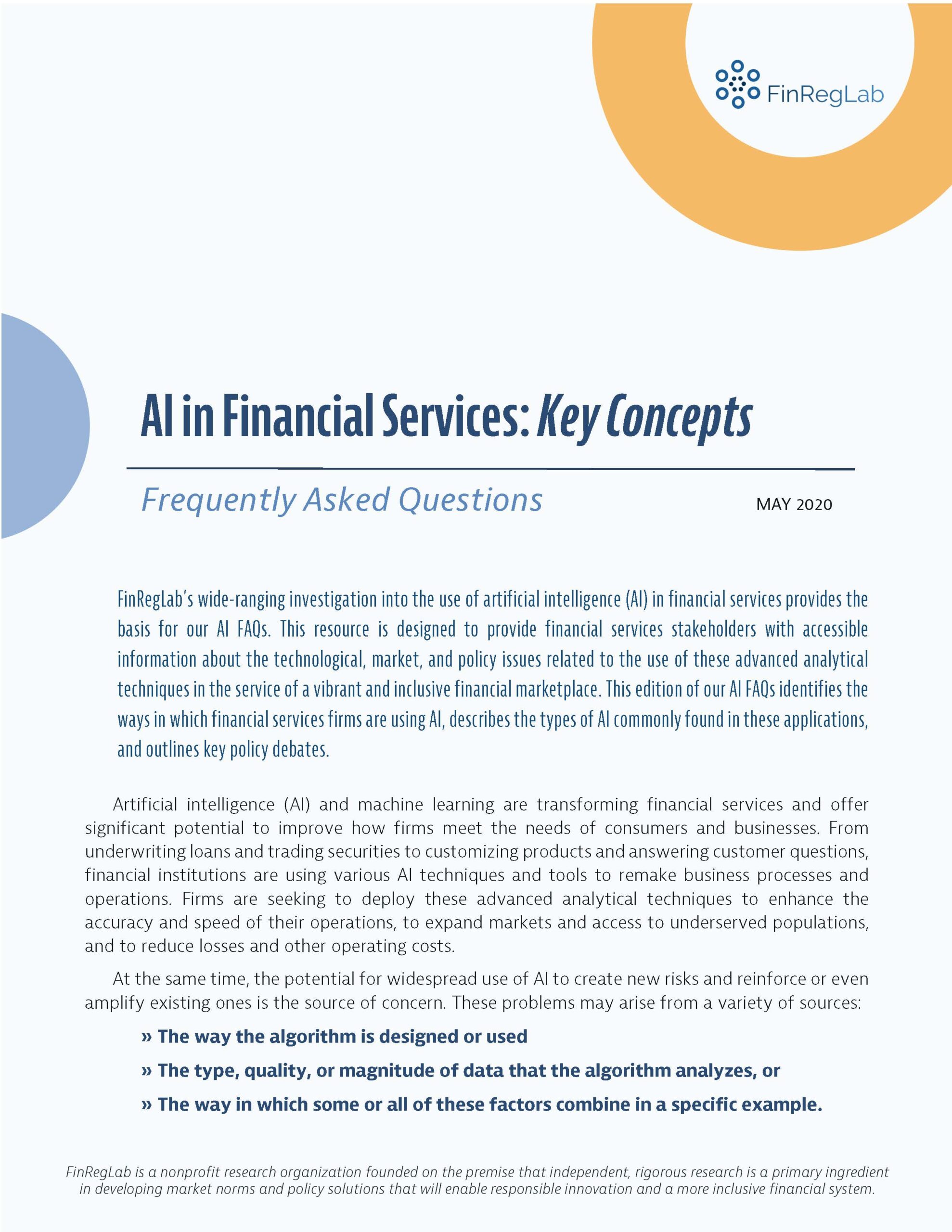
What are artificial intelligence (AI) and machine learning?
Artificial intelligence (AI) is a term coined in 1956 to describe computers that perform processes or tasks that “traditionally have required human intelligence.”1 We routinely rely on AI in our daily lives—when we search for content on the internet, use social media, or communicate through messaging platforms. Automated processes have long been used and trusted in very sensitive applications—like commercial aviation, where pilots report spending seven minutes or less manually piloting their aircraft, primarily during takeoff and landing.2 Advances in modelling and data science are pushing AI to the fore in high-stakes uses, such as diagnosing cancer,3 matching available organs to those in need of transplants,4 and enabling self-driving cars.5
Machine learning refers to the subset of artificial intelligence that gives “computers the ability to learn without being explicitly programmed.”6 Even the programmer may not understand the underlying processes by which the model operates. His or her role is to provide the learning algorithm a sufficient number of examples to derive a process or processes for predicting an outcome. Machine learning is a way to build mathematical models that can be used in a variety of contexts. In some cases, like fraud screening and credit underwriting, the model’s output is used to inform a particular decision: approve or deny an application or transaction. In others, the models help to enable broader AI-driven processes—like enabling a self-driving car, a robo-advisor, or a chatbot.
How different are AI and machine learning from other common forms of predictive modelling?
Machine learning and other common forms of predictive modelling including logistic regression share some of the same goals: to make accurate predictions derived from data given uncertainties inherent in the calculations.
Neither AI nor machine learning are new. Both draw on the same bodies of knowledge and experience as other forms of predictive modelling—mathematics, statistics, and computer science. In the 1930s, Alan Turing’s description of stored-program machines—now known widely as Turing machines—marks the first modern work on computing and included machine learning concepts. AI began to be studied intensively in Defense Department research in the 1950s, but did not lead to widespread adoption.
The confluence of dramatic increases in computing power and exponential growth of digital data in the 1990s has brought machine learning back to the forefront of research and development. As a result, machine learning techniques that have long been known are being operationalized across the economy and in financial services. The shift from classical AI research to the contemporary development of machine learning focuses on structuring intelligence tasks as statistical learning problems rather than trying to create a program based on how a human might approach such tasks.
As firms drive to adopt models that are more complex than what they replace, the complexity of these new approaches requires reconsideration of a range of strategic and operational issues, including how well they comply with the requirements of applicable regulatory and risk management frameworks. This has, in turn, spurred development of new machine learning modelling techniques and tools, especially in the area of model interpretability or explainability.
However, from the point of view of practitioners trying to implement and use new analytical methods at scale, the effort to differentiate AI and machine learning from incumbent forms of predictive modelling may be not be particularly meaningful. What may look from some perspectives like a single model may actual be a suite of models that combines machine learning and logistic regression and other more traditional modelling techniques. Any one model builder may choose from a diverse, varied, and rapidly-evolving set of modeling techniques based on the specific needs of his or her use case, subject to applicable resource, business process, and regulatory limitations.
How can we evaluate a specific use of AI or machine learning to understand relevant differences when compared to incumbent models?
Even if data scientists and model builders see substantial continuity between AI and prior forms of statistical prediction, some questions—especially those about how to interpret and apply existing legal, regulatory, and risk management frameworks—do require understanding the differences between AI models and the incumbent models they might replace. The following questions can focus attention on the differences relevant in this context:
- Are the risks related to a particular use of AI or machine learning the result of the model, the data being analyzed by the model, or some combination of the two?
- Does a particular use of AI or machine learning introduce novel risks?
- Does a particular use of AI or machine learning change or accentuate risks associated with traditional forms of statistical prediction doing the same thing?
- How well do existing law, regulation, and risk management processes enable effective oversight of these risks?
In this context, it is worth remembering that like many earlier waves of new mathematics and technology, much of AI’s potential benefit derives from its ability to do something—detect patterns and correlations—that humans cannot do as well themselves. But humans are still responsible for critical decisions about model governance and need to be able to understand how and why models produce the results they do. Given that AI models are often more complex than their predecessors, data scientists, academics, and industry practitioners are now focused intensely on developing more interpretable machine learning models as well as separate tools that can be paired with “black box” AI models to enable more oversight.
This emphasis on the ability to explain AI models points to a critical question for widespread adoption across use cases and regulatory frameworks: how can we operate machine learning models with sufficient insight into their functioning to be able to detect errors and problems, manage risks, and explain specific predictions?
How are AI models different from other forms of automated prediction?
Traditional forms of automated prediction often use computers to make computations, but they also typically rely on programmers to define the basic relationships between the inputs and target variable. By contrast, machine learning algorithms tend to be given only the target variable and then use computationally intensive processes to identify the relationships between various data inputs and the target variable and produce the predictive model. The machine learning algorithm has the capacity to change computational processes and improve the model’s performance at each step of the process.
In this regard, the development of traditional automated prediction models can be thought of as being more dependent on the programmer or model builder. That in turn means that the machines operate with less flexibility and that the resulting models are limited by the relationships between the data and the prediction that the programmer can perceive and program.
By contrast, AI-driven methods give the machine more flexibility to create code based on the analysis of data, often in volumes and at speeds well beyond what would be manageable for a human. This often produces significantly more complex models that are more difficult for humans to understand and monitor, whatever their specific benefits might be. Those differences are important—both for how users and their regulators evaluate the relative benefits of AI in particular applications and adapt and operationalize frameworks to govern the associated risks.
Nevertheless, it is worth questioning whether those differences are as defining as they can seem. The term AI in particular often conjures up a dystopian future that science fiction has long explored: robots gone awry and loss of agency that challenges our sense of what it means to be human.7 In practice, the forms of AI and machine learning being used by firms across the economy today may represent a far less daunting change, especially in areas like financial services where their development and use will be subject to extensive regulation and oversight.
Approaches to managing automated models are well established in financial services, although firms and policymakers are still evaluating whether and how those need to be adapted for use with AI models.
How are machine learning models developed?
Although the intensity of individual steps can vary, machine learning models are generally developed in the following steps:
- Algorithm Selection: The model builder picks a learning algorithm or algorithms based on the application for which the model is being developed. Tradeoffs between algorithm types include the amount of data required, the types of relationships they can look for, and the ease of providing explanations of how the model works and for specific results.
- Training: Training data is fed into a learning algorithm that is designed to produce a predictive model based on the information contained in the data selected for use in training. Compared to traditional statistical modelling techniques, the machine learning algorithm rather than a human coder determines the structure of the resulting model.
- Validation: After training, predictive models evaluate a hold-out data set—one other than the one on which it was trained—to evaluate its reliability and robustness. Validation is particularly important in building machine learning models given the risk of overfitting— the risk that the machine learning algorithm fits the predictive model too narrowly to the specific characteristics of limited training data, which may result in unnecessary complexity and increase the fragility of the model’s performance.
- Tuning: Machine learning models are then “tuned” in order to maximize performance based on validation and testing results. Tuning, validation, and testing may occur in several iterations during model development. Tuning is a critical step to reduce overfitting problems. Regularization is one technique used to tune a model—here, an additional term is added to constrain the model so that specific coefficients cannot take extreme values. Hyperparameters are another tuning tool. These metrics or knobs to adjust models are set before training begins either by a data scientist or autoML software and their values can be changed during tuning.
- Testing: Testing involves statistically assessing performance using data that neither the data scientist nor model have seen.
- Shadow deployment: Firms typically run the developmental model parallel to models that are already in production. This permits direct comparison to incumbent models on performance, stability, and other metrics relevant to the use case and refinement of model design, implementation, and risk management plans.
How do AI and machine learning models change after deployment?
Once in use, certain kinds of AI and machine learning models may refine the processes by which predictions are made based on analyses of new data and information, including prior performance of its predictions. The refinements can include incorporation of new variables created through complex computational processes, changing the weight given to variables in response to new conditions, and excluding variables where appropriate. The degree to which machine learning models change while in use, as well as the volume and nature of data being used for analysis, varies significantly based on the context in which the model is being used and the specific machine learning techniques being used. In most cases, model retraining and testing will be done offline, and updates deployed only after the relevant performance criteria have been met.
What are supervised learning, unsupervised learning, and reinforcement learning?
Financial services applications of AI disproportionately use machine learning, which uses the following forms of learning:
- Supervised learning: Supervised learning refers to a model that is trained on a data set that includes an outcome measure for its target variable.8 For example, if the data set that is used to train a fraud model contains an indication that specifies the subset of transactions that turned out to be fraudulent, the resulting model is a supervised learning model. Supervised learning is the most common approach within financial services, especially in areas like credit scoring and underwriting.
- Unsupervised learning: An unsupervised learning model detects patterns in a data set that does not include the outcome measure for its target variable. In the example above, a fraud model would be unsupervised learning if the training data did not identify which transactions proved to be fraudulent. The patterns detected can be used directly by the model to make predictions or as features in a supervised learning model.9 Unsupervised learning is commonly used for anomaly detection and in use cases like genetic testing.
- Reinforcement learning: Where reinforcement learning is used, the model is trained on data, identifies an action for each variable, and receives input from a human or another model that helps the algorithm learn.10 This includes learning to make a series of decisions correctly—such as playing and winning games.
What is deep learning?
Deep learning is a form of machine learning that was inspired by the analytical processes of the human brain and that uses multiple layers to progressively extract deeper meaning from the input data. Neural networks consisting of at least four or five analytical layers are a common example of deep learning. Deep learning, which can be used in the context of supervised, unsupervised, or reinforcement learning, is being used to help self-driving cars detect objects such as stop signs and traffic lights.
How are AI and machine learning being used in financial services?
Financial institutions are already using AI and machine learning in a variety of contexts, as well as exploring potential additional applications. These uses include:
- Fraud and financial crimes detection: AI and machine learning have enormous power to improve risk detection in areas where data-intensive, iterative processes are needed to identify individual illicit acts based on patterns within massive volumes of streaming activity. Financial institutions have long leveraged this capability to screen applications and monitor transactions for fraud. These advanced analytical processes deliver more accurate risk identification and reduce the number of applications or transactions improperly rejected as false positives. In this context, emerging forms of machine learning can enable sharing of insights among firms and between regulatory and law enforcement agencies without sharing any data that is legally protected or sensitive and undermining compliance with privacy and data localization laws.
- Securities trading: Machine learning and other forms of artificial intelligence are widely used in securities markets. Here, machine learning has increased the speed and volume at which trading occurs, allowing market participants to identify and capture gains from smaller pricing gaps than previously possible.
- Credit scoring and underwriting: Lenders and third party-credit score providers are evaluating various ways in which machine learning can improve the predictive power of the models they use to evaluate default risk with the aim of improving predictive performance. Some firms use data inputs derived from machine learning into more traditional models or use machine learning models to assess traditional underwriting data.
- Customer segmentation: Financial services firms, like firms across the economy, use machine learning techniques to develop targeted marketing and shape the contents and terms of product offers.
- Product / content recommenders and robo-advisors: Financial services firms, like most firms conducting business online, use machine learning to create software tools that generate and provide customized suggestions for products, services, or other content that might be of interest. Robo-advisors are a more sophisticated form of recommendation systems.
- Identity verification: Financial institutions verify and authenticate the identity of applicants and customers to comply with anti-financial crimes requirements, prevent fraud, and ensure they are obtaining accurate information about applicants and customers. Advanced analytical processes help firms approve all legitimate applicants and customers with minimal disruption in the customer experience.
- Chatbots and virtual assistants: A chatbot or virtual assistant helps customers solve problems or make transactions. In various contexts, a customer can ask for information and respond to inquiries and statements from the chatbot using natural language in text or audio. Firms in financial services and more broadly use this technology for customer relationship management.
With respect to each of these contexts, AI may improve the predictiveness of models over time when compared to existing methods, because it can harvest insight from significantly greater volumes of data and quickly adjust calculations and predictions based on changes in that data. In applications that can be largely manual for some firms, like responding to customer questions and identity verification, machine learning may also improve the consistency of results and help reduce operating costs.
Further Reading
Majid Bazarbash, FinTech in Financial Inclusion: Machine Learning Applications in Assessing Credit Risk, International Monetary Fund (May 17, 2019), available at https://www.imf.org/en/Publications/WP/Issues/2019/05/17/FinTech-in-Financial-Inclusion-Machine-Learning-Applications-in-Assessing-Credit-Risk-46883
Financial Stability Board, Artificial Intelligence and Machine Learning in Financial Services (November 1, 2017), available at https://www.fsb.org/wp-content/uploads/P011117.pdf
What are the key policy debates about using AI in financial services?
Data scientists, academics, industry practitioners, advocates, and policymakers are all turning their attention to resolving uncertainty about when we can trust AI and when we cannot. This challenge is all the more complex in the financial sector, where extensive regulatory frameworks force consideration of questions about trustworthiness more holistically and at an earlier stage than may occur in other sectors. Indeed, implementing machine learning in the financial system often requires meeting exacting requirements focused on securing the financial system from illicit activity, promoting responsible risk-taking, and providing customers broad, non-discriminatory
In the financial services context, trustworthiness speaks broadly to the following components, which apply broadly to all forms of statistical prediction including AI and machine learning techniques:
- Reliability: Firms interested in adopting machine learning need to demonstrate that a particular model performs as intended for its use case—that is, the model’s predictions meet its needs for accuracy—just as incumbent models do.
- Robustness: A second aspect of performance relates to how well the model makes predictions in unexpected conditions. In the context of machine learning, the need to consider how a particular model reacts when confronted with changes in the data environment in which it will be deployed is especially important. Machine learning models may not do well in recognizing changes in the data pipeline and return lower quality predictions when confronted with data that is different than that on which they trained, even though they may offer users the ability to recalibrate models faster than other forms of automated prediction.
- Explainability: Addressing “black box” functionality of certain AI technologies is critical to financial services, where firms have to respond to specific regulatory requirements, such as demonstrating general model performance and explaining specific decisions. Global explainability refers to the ability to understand the high-level decision-making processes used by a model and is relevant to evaluating a model’s overall behavior and fitness for use. Local explainability speaks to the ability to identify the basis for specific decisions directed by the model. Both forms of explainability are important to enable appropriate human learning from and oversight of AI and machine learning models in financial services contexts.
- Privacy: Machine learning can dramatically improve our systemic ability to extract information from data sets, including data sets that are exponentially larger and more diverse than previously used for particular financial services functions. This raises questions about individuals’ ability to limit the use of certain kinds of information and firms’ decisions to use particular kinds of data, and methods for obtaining data.
- Security: Established frameworks may need to evolve to secure AI models since they can be manipulated without direct access to their code by, for example, maliciously em- bedding signals in social network feeds or news feeds that are not detectable by humans. Further, because machine learning models encode aspects of training data into the mechanisms by which they operate, they have the potential to expose private or sensitive information from the training data to users.
- Fairness: Whether and how AI and machine learning can be used in compliance with non- discrimination requirements are foremost among a range of fairness questions related to the use of AI. Particularly in underwriting and marketing contexts, the shift to machine learning models creates concern about our ability to prevent use of race, gender, or other protected characteristics. For example, machine learning models may be able to derive race, gender, or other protected class information by analyzing patterns in the input data and can then factor information that is barred from use into its analysis. The power of machine learning to enable assessments at a much more personal level may also intensify critiques of risk-based pricing. Other concerns involve broader notions of fairness, such as factoring in types of information that do not have an obvious relationship to the prediction or that the data subjects cannot control.
Further Reading
Umang Bhatt, Alice Xiang, Shubham Sharma, Adrian Weller, Ankur Taly, Yunhan Jia, Joydeep Ghosh, Ruchir Puri, José M.F. Moura, & Peter Eckersley, Explainable Machine Learning in Deployment (2019), available at https://arxiv.org/pdf/1909.06342.pdf.
Jon Kleinberg, Jens Ludwig, Sendhil Mullainathan, & Cass Sunstein, Discrimination in the Age of Algorithms, 10 J. of Legal Analysis (2018).
Cynthia Rudin, Stop Explaining Black Box Machine Learning Models for High Stakes Decisions and Use Interpretable Models Instead, Nature Machine Intelligence (Sept. 22, 2019).
Andrew D. Selbst & Solon Barocas, The Intuitive Appeal of Explainable Machines, 87 Fordham L. Rev. 1085 (2018).
David Spiegelhalter, Should We Trust Algorithms?, Harvard Data Sci. Rev. (Jan. 31, 2020).
Harini Suresh & John V. Guttag, A Framework for Understanding Unintended Consequences of Machine Learning (Feb. 17, 2020), available at https://arxiv.org/pdf/1901.10002.pdf.
What forms of AI and machine learning are most commonly used in financial services? How do they work?
Firms are still evaluating potential uses for varied forms of AI and machine learning—and even how to pair different forms of these modelling techniques with each other and with traditional statistical prediction methods. The following represent forms of advanced modelling techniques that are most relevant to the financial system:
- Decision trees and forests: Decision trees implement traditional “if – then” logic by converting a series of uncertain events relevant to an outcome that the model will predict into a series of binary decisions in a hierarchical structure. They visually represent their partitioning decision steps by each variable included in the algorithm, showing how each additional variable contributes to an ultimate decision. The decisions split based on variables in each branch.11 So, in credit underwriting, a decision tree might be designed to evaluate the likelihood that a particular applicant will pay back a loan. The tree would start with one variable and branch out to other variables with questions like: How often is this person late on bills? What portion of their income is rent or mortgage? How much do they pay for a car loan? Do they have over 30 percent credit card usage? Each of these inquiries branch off from each other, forming a string of calculations that can be used to predict an answer the question above. Individual decision trees or groups of decision trees can be used to create a model. Random forest models and gradient boosting machines are examples of ensemble models using multiple decision trees. Decision trees are well suited to credit risk assessment, customer loyalty programs, and fraud detection.
- Neural networks: Neural networks are a form of deep learning that emulate human brain learning to discover complex patterns in data.12 They do so by evaluating data in a sequence of layers designed to recognize, analyze, and connect different features of the data set, which are designed to resemble neurons working in the background of the human mind. There is then an output produced, from which humans can correct any issues in the data analysis and the machine will continue to learn, eventually not needing human help to fix errors. In credit scoring, neural networks may be particularly effective in tailoring weights of different variables to each personal situation, factoring in improvement over time in repaying debt rather than just looking at overall debt ratio, for example.13 Neural networks are generally the most difficult “black box” model to explain because they produce the most complex modeling functions, although new techniques like integrated gradients approaches are improving their interpretability.14 Neural networks have long been used in fraud screening, and may be used by credit card issuers in risk scoring models.15
Further Reading
Amina Adadi & Mohammed Berrada, Peeking Inside the Black-Box: A Survey of Explainable Artificial Intelligence (XAI), 6 IEEE Access (September 17, 2018).
Bazarbash (2019).
Patrick Hall, On the Art and Science of Explainable Machine Learning: Techniques, Recommendations, and Responsibilities (August 2, 2019), available at https://arxiv.org/pdf/1810.02909.pdf.
Joel Vaughan, Agus Sudjianto, Erind Brahimi, Jie Chen, & Vijayan N. Nair, Explainable Neural Networks based on Additive Index Models (June 2018), available at https://arxiv.org/abs/1806.01933.
What is the basis for believing that machine learning could improve credit underwriting?
Credit score developers and lenders are exploring the use of machine learning techniques to improve their ability to predict credit risk with or without new data sources. Machine learning has the potential to improve the speed and accuracy of predictions and to improve our ability to infer the entire distribution of potential outcomes and understand the variability of model predictions. These benefits, if realized, would serve goals broadly shared by borrowers, firms, policymakers, and investors alike:
- Assess the risk of default of and price offers of credit to individual applicants in more personalized ways
- Reduce mispricing based on inaccurate estimation of the likelihood of default
- Reduce default rates and losses
- Enhance the efficiency of lending markets
- Improve risk assessment for those who lack sufficient credit history to be scored using traditional models and data sources16
Individual firms are at different points in the process of exploring using machine learning for credit underwriting depending on their strategy, resources, legal structure, comfort level with unresolved policy questions, and other factors. Any of the following scenarios may be relevant, but each poses different benefits and risks for financial inclusion:
- Machine learning model analyzes traditional underwriting inputs: Compared to a traditional regression model, a machine learning underwriting model, using exactly the same information as regression models do today, has the potential to derive superior predictive performance from an applicant’s information.17
- Machine learning model also analyzes alternative financial data as an under- writing input: Adding financial data not traditionally included in underwriting analyses—such as transaction and payments account data—can improve the predictive power of traditional underwriting models.18 Incorporating recurring periodic payments, such as rent, utility, and mobile phone payments, and basic cash-flow metrics, such as average monthly transaction account cushion, may further enhance the benefits provided by use of machine learning on traditional underwriting inputs.
- Machine learning model also analyzes “big data” as an underwriting input: The most aggressive use of data for credit underwriting would include an array of non- financial data, such as social media data and behavioral data of various kinds (e.g., internet search histories or the content, time, and location of purchases). Whether incorporating this broader data into traditional underwriting models responsibly expands access to credit is not well understood. In this scenario, machine learning models may be able to derive meaningful credit insights from a vast and disparate constellation of data points, but the potential benefits, especially for financial inclusion, are more difficult to predict given privacy, bias, and fairness questions raised by using some forms of data for underwriting.
More research is needed to understand the financial inclusion benefits, as well as the risks of discrimination, unfairness, or inaccurate prediction, that go along with each of these scenarios.
Further Reading
Adadi & Berrada (2018).
Bazarbash (2019).
Amir Ehsan Khandani, Adlar Kim, & Andrew Lo, Consumer Credit-Risk Models via Machine Learning Algorithms, 34 Journal of Banking and Finance (2010).
Maria Fernandez Vidal & Fernando Barbon, Credit Scoring in Financial Inclusion: How to use advanced analytics to build credit-scoring models that increase access, Consultative Group to Assist the Poor (July 2019), available at https://www.cgap.org/sites/default/files/publications/2019_07_Technical_Guide_CreditScore.pdf.
Andreas Fuster, Paul Goldsmith-Pinkham, Tarun Ramadorai, & Ansgar Walther, Predictably Unequal? The Effects of Machine Learning on Credit Markets (March 11, 2020), available at https://papers.ssrn.com/sol3/papers.cfm?abstract_id=3072038.
Luke Merrick & Ankur Taly, The Explanation Game: Explaining Machine Learning Models with Cooperative Game Theory (September 17, 2019), available at https://arxiv.org/abs/1909.08128.
Endnotes
[1] Financial Stability Board, Artificial Intelligence and Machine Learning in Financial Services (November 1, 2017), available at https://www.fsb.org/wp-content/uploads/P011117.pdf; Ting Huang, Brian McGuire, Chris Smith, & Gary Yang, The History of Artificial Intelligence, University of Washington (December 2006), available at https://courses.cs.washington.edu/courses/csep590/06au/projects/history-ai.pdf.
[2] John Markoff, Planes Without Pilots, N.Y. Times (Apr. 6, 2015), available at https://www.nytimes.com/2015/04/07/science/planes- without-pilots.html?_r=0.
[3] Neil Savage, How AI Is Improving Cancer Diagnostics, Nature.com (March 25, 2020), available at https://www.nature.com/articles/d41586-020-00847-2.
[4] Sachin Waikar, Blog, Evolution of an Algorithm: Lessons from the Kidney Allocation System, Stanford Institute for Human-Centered Artificial Intelligence (2020), available at https://hai.stanford.edu/blog/evolution-algorithm-lessons-kidney-allocation-system.
[5] Aarian Marshall, Teaching Self-Driving Cars to Watch for Unpredictable Humans, Wired.com (Dec. 4, 2019), available at https://www.wired.com/story/teaching-self-driving-cars-watch-unpredictable-humans/.
[6] Financial Stability Board, Artificial Intelligence and Machine Learning in Financial Services (2017); see also Arthur L. Samuel, “Some Studies in Machine Learning Using the Game of Checkers,” IBM Journal 211-229 (1959); Tom Mitchell, Machine Learning, New York: McGraw Hill (defining machine learning as the “field of study that gives computers the ability to learn without being explicitly programmed”); Michael Jordan and Tom Mitchell, “Machine learning: Trends, perspectives, and prospects,” 349 Science (2015) (defining machine learning as “the question of how to build computers that improve automatically through experience”).
[7] 2001: A Space Odyssey (1968) – I’m Sorry, Dave Scene (3/6) | Movieclips, YouTube (Jan. 31, 2019), available at https://www.youtube.com/watch?v=Wy4EfdnMZ5g; David Leslie, Raging Robots, Hapless Humans: The AI Dystopia, Nature.com (Oct. 2, 2019), available at https://www.nature.com/articles/d41586-019-02939-0.
[8] Majid Bazarbash, FinTech in Financial Inclusion: Machine Learning Applications in Assessing Credit Risk, International Monetary Fund (May 17, 2019), available at https://www.imf.org/en/Publications/WP/Issues/2019/05/17/FinTech-in-Financial-Inclusion-Machine- Learning-Applications-in-Assessing-Credit-Risk-46883; Financial Stability Board, Artificial intelligence and machine learning in financial services (November 1, 2017).
[9] Ibid.
[10] Financial Stability Board, Artificial intelligence and machine learning in financial services (November 1, 2017).
[11] Yan-yan Song & Ying Lu, Decision Tree Methods: Applications for Classification and Prediction, 27 Shanghai Archives of Psychiatry (April 25, 2015).
[12] Radoslaw M. Cichy and Daniel Kaiser, Deep Neural Networks as Scientific Models, 23 Trends in Cognitive Science (April 1, 2019).
[13] David West, Neural Network Credit Scoring Models, 27 Computers and Operations Research (Sept. 2000).
[14] Joel Vaughan, Agus Sudjianto, Erind Brahimi, Jie Chen, & Vijayan N. Nair, Explainable Neural Networks based on Additive Index Models (June 5, 2018), available at https://arxiv.org/abs/1806.01933.
[15] Office of the Comptroller of the Currency, Credit Card Lending Version 1.2, Comptroller’s Handbook 14, 46 (January 6, 2017), available at https://www.occ.treas.gov/publications-and-resources/publications/comptrollers-handbook/files/credit-card-lending/index-credit- card-lending.html.
[16] FinRegLab, The Use of Cash-Flow Data in Credit Underwriting: Market Context & Policy Analysis 8-12 (2020); Consumer Financial Protection Bureau, Kenneth P. Brevoort, Philipp Grimm, & Michelle Kambara, Data Point: Credit Invisibles, Consumer Financial Protection Bureau 4-6 (May 2015), available at https://files.consumerfinance.gov/f/201505_cfpb_data-point-credit-invisibles.pdf; Peter Carroll and Saba Rehmani, Alternative Data and the Unbanked, Oliver Wyman (2017), available at https://www.oliverwyman.com/content/dam/oliver-wyman/v2/publications/2017/may/Oliver_Wyman_Alternative_Data.pdf.
[17] Andreas Fuster, Paul Goldsmith-Pinkham, Tarun Ramadorai, & Ansgar Walther, Predictably Unequal? The Effects of Machine Learning on Credit Markets (March 11, 2020), available at SSRN: https://ssrn.com/abstract=3072038 or http://dx.doi.org/10.2139/ssrn.3072038.
[18] FinRegLab, The Use of Cash-Flow Data in Underwriting Credit: Empirical Research Findings (July 2019), available at https://finreglab.org/wp-content/uploads/2019/07/FRL_Research-Report_Final.pdf.
Related Publications
-
Explainability & Fairness in Machine Learning for Credit Underwriting: Policy Analysis
This policy analysis explores the regulatory and public policy implications of the increasing use of machine learning models and explainability and fairness techniques for credit underwriting in depth, particularly for model risk management, consumer disclosures, and fair lending compliance.
-
Explainability & Fairness in Machine Learning for Credit Underwriting: Policy & Empirical Findings Overview
This paper summarizes the machine learning project’s key empirical research findings and discusses the regulatory and public policy implications to be considered with the increasing use of machine learning models and explainability and fairness techniques.
-
Machine Learning Explainability & Fairness: Insights from Consumer Lending
This empirical white paper assesses the capabilities and limitations of available model diagnostic tools in helping lenders manage machine learning underwriting models. It focuses on the tools’ production of information relevant to adverse action, fair lending, and model risk management requirements.
-
The Use of Machine Learning for Credit Underwriting: Market & Data Science Context
This report surveys market practice with respect to the use of machine learning underwriting models and provides an overview of the current questions, debates, and regulatory frameworks that are shaping adoption and use.
-
AI FAQS: The Data Science of Explainability
This fourth edition of our FAQs focuses on emerging techniques to explain complex models and builds on prior FAQs that covered the use of AI in financial services and the importance of model transparency and explainability in the context of machine learning credit underwriting models.
-
Explainability and Fairness in Machine Learning for Credit Underwriting
FinRegLab worked with a team of researchers from the Stanford Graduate School of Business to evaluate the explainability and fairness of machine learning for credit underwriting. We focused on measuring the ability of currently available model diagnostic tools to provide information about the performance and capabilities of machine learning underwriting models. This research helps stakeholders… Learn More
About FinRegLab
FinRegLab is an independent, nonprofit organization that conducts research and experiments with new technologies and data to drive the financial sector toward a safe and responsible marketplace. The organization also facilitates discourse across the financial ecosystem to inform public policy and market practices. To receive periodic updates on the latest research, subscribe to FRL’s newsletter and visit www.finreglab.org. Follow FinRegLab on LinkedIn.
FinRegLab.org | 1701 K Street Northwest, Suite 1150, Washington, DC 20006