Publications
Explainability & Fairness in Machine Learning for Credit Underwriting: Policy & Empirical Findings Overview
Overview Paper
Report Summary
This overview summarizes our empirical findings and describes emerging policy dialogues across several regulatory areas as lenders, advocates, policymakers, and stakeholders consider differences between the information and techniques used to develop, manage, and validate traditional underwriting models as compared to machine learning models.
It draws on the discussions of three policy working groups that FinRegLab convened in 2022 as well as other interviews, convenings, and analysis. The paper highlights topics on which regulatory guidance could encourage more consistent, responsible use of machine learning models and explainability and fairness techniques, even as the underlying technologies and research are continuing to evolve.
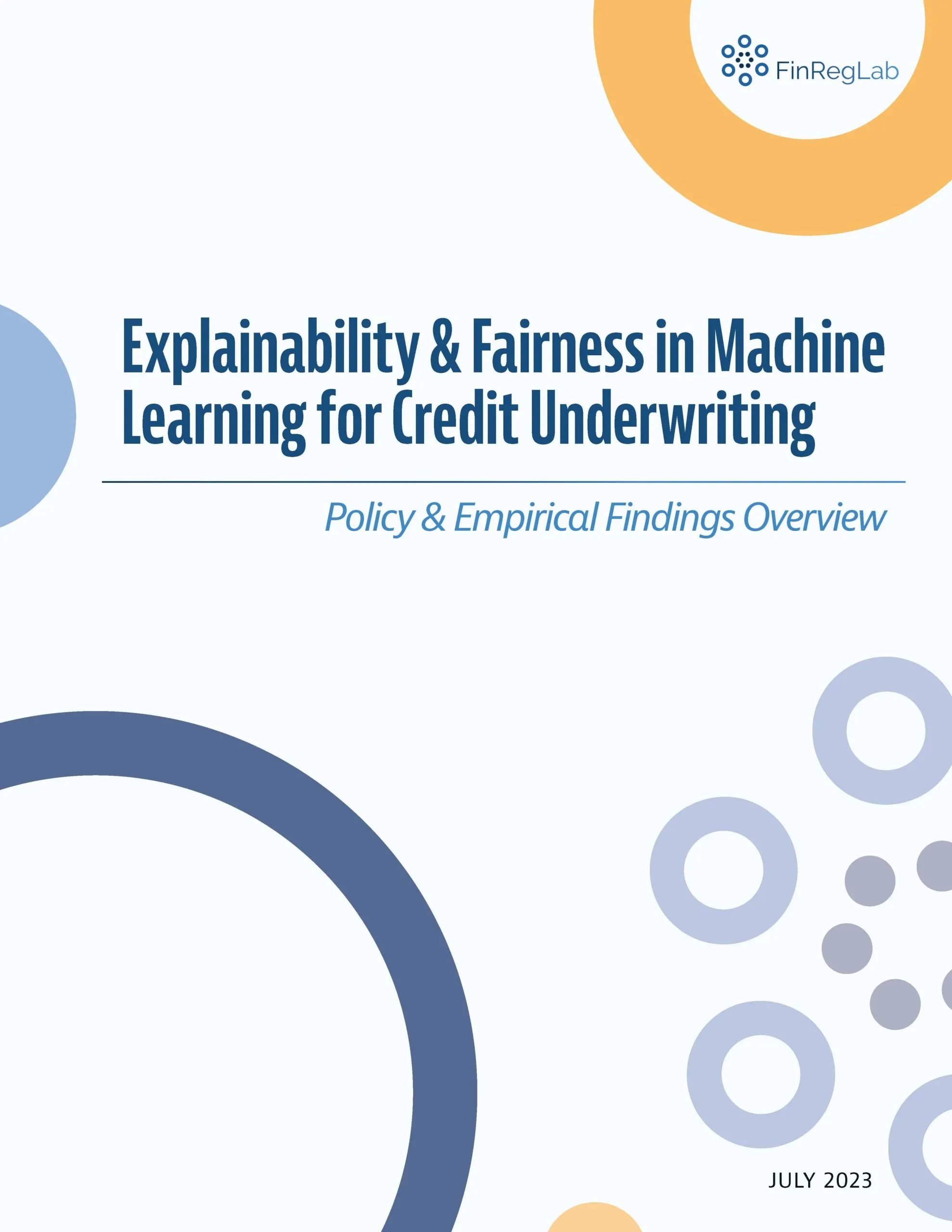
Acknowledgments
FinRegLab would like to recognize the presenters and members of our project Advisory Board and policy working groups who contributed to productive discussion of the development of the design, execution, and interpretation of this research and the policy insights reflected in this document. The Advisory Board and policy working groups consisted of subject matter experts from computer science, economics, financial services, and regulatory backgrounds and included representatives from approximately 45 major institutions including bank and nonbank financial institutions, technology firms, advocacy and civil society organizations, and academic institutions. State and federal regulators participated as observers in Advisory Board and policy working group meetings.
We would like to thank the following individuals who provided feedback on this report:
We would also like to acknowledge the FinRegLab team who worked on various elements of the research project:
With Support From
Mastercard Center for Inclusive Growth
The Mastercard Center for Inclusive Growth advances equitable and sustainable economic growth and financial inclusion around the world. The Center leverages the company’s core assets and competencies, including data insights, expertise, and technology, while administering the philanthropic Mastercard Impact Fund, to produce independent research, scale global programs and empower a community of thinkers, leaders, and doers on the front lines of inclusive growth. The Center has provided funding to support this research.
JPMorgan Chase & Co.
(NYSE: JPM) is a leading financial services firm based in the United States of America (“U.S.”), with operations worldwide. JPMorganChase had $4.4 trillion in assets and $351 billion in stockholders’ equity as of March 31, 2025. The Firm is a leader in investment banking, financial services for consumers and small businesses, commercial banking, financial transaction processing and asset management. Under the J.P. Morgan and Chase brands, the Firm serves millions of customers in the U.S., and many of the world’s most prominent corporate, institutional and government clients globally. Information about JPMorgan Chase & Co. is available at www.jpmorganchase.com.
Flourish Ventures
Flourish, a venture of the Omidyar Group, has provided operating support to FinRegLab since its inception. Flourish is an evergreen fund investing in entrepreneurs whose innovations help people achieve financial health and prosperity. Established in 2019, Flourish is funded by Pam and Pierre Omidyar. Pierre is the founder of eBay. Managed by a global team, Flourish makes impact-oriented investments in challenger banks, personal finance, insurtech, regtech, and other technologies that empower people and foster a fairer, more inclusive economy.
Related Publications
-
Machine Learning Explainability & Fairness: Insights from Consumer Lending
This empirical white paper assesses the capabilities and limitations of available model diagnostic tools in helping lenders manage machine learning underwriting models. It focuses on the tools’ production of information relevant to adverse action, fair lending, and model risk management requirements.
-
The Use of Machine Learning for Credit Underwriting: Market & Data Science Context
This report surveys market practice with respect to the use of machine learning underwriting models and provides an overview of the current questions, debates, and regulatory frameworks that are shaping adoption and use.
-
Explainability and Fairness in Machine Learning for Credit Underwriting
FinRegLab worked with a team of researchers from the Stanford Graduate School of Business to evaluate the explainability and fairness of machine learning for credit underwriting. We focused on measuring the ability of currently available model diagnostic tools to provide information about the performance and capabilities of machine learning underwriting models. This research helps stakeholders… Learn More
Events
-
Getting Ahead of the Curve: Emerging Issues in the Use of Artificial Intelligence and Machine Learning in Credit Underwriting
Read more: Getting Ahead of the Curve: Emerging Issues in the Use of Artificial Intelligence and Machine Learning in Credit UnderwritingFinRegLab hosted a webinar with senior federal financial regulators in January 2024 to discuss the growing use of artificial intelligence and machine learning in financial services, including credit underwriting.
-
Machine Learning Explained: Evidence on the Explainability and Fairness of Machine Learning Credit Models
Read more: Machine Learning Explained: Evidence on the Explainability and Fairness of Machine Learning Credit ModelsFinRegLab hosted a virtual conference in April 2022 featuring research being conducted by FinRegLab and Professors Laura Blattner and Jann Spiess of the Stanford Graduate School of Business on the use of machine learning in credit underwriting, with a particular focus on their potential implications for explainability and fairness.
About FinRegLab
FinRegLab is an independent, nonprofit organization that conducts research and experiments with new technologies and data to drive the financial sector toward a safe and responsible marketplace. The organization also facilitates discourse across the financial ecosystem to inform public policy and market practices. To receive periodic updates on the latest research, subscribe to FRL’s newsletter and visit www.finreglab.org. Follow FinRegLab on LinkedIn.
FinRegLab.org | 1701 K Street Northwest, Suite 1150, Washington, DC 20006